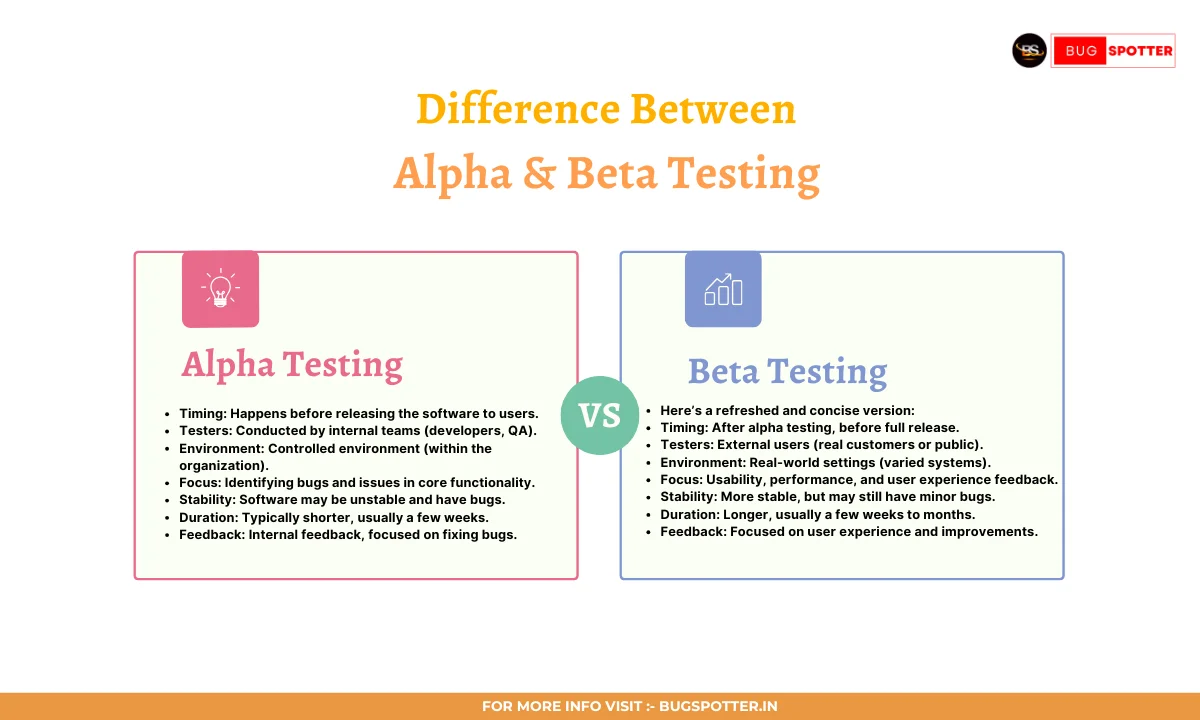
In the world of data analysis, working with large datasets can often be time-consuming, resource-intensive, and impractical. Fortunately, sampling methods allow data analysts to work with a smaller subset of data that accurately represents the entire population. Whether you’re conducting surveys, analyzing trends, or building predictive models, understanding sampling techniques is essential. In this blog post, we will explore the different sampling methods, why they are important, and how they help data analysts derive meaningful insights from limited data.
Sampling is the process of selecting a subset of data from a larger population or dataset. This subset, known as the sample, is chosen in such a way that it represents the characteristics of the whole population. Sampling is critical when the entire population is too large or expensive to analyze in full. By studying a representative sample, data analysts can draw conclusions and make predictions without having to analyze every data point.
Sampling methods are essential for several reasons:
The key to successful sampling is ensuring that the sample accurately represents the population, which is why choosing the right sampling method is crucial.
There are two main categories of sampling methods: Probability Sampling and Non-Probability Sampling. Let’s explore these in detail.
In probability sampling, every individual in the population has a known, non-zero chance of being selected. This method ensures that the sample is representative and allows for statistical analysis, such as estimating population parameters and testing hypotheses. There are several types of probability sampling:
Â
In non-probability sampling, not every individual has a known or equal chance of being selected. These methods are generally less accurate and are used when random sampling is not feasible or when the goal is to explore trends rather than make generalizations about the population.
Â
In probability sampling, every member of the population has a known, non-zero chance of being selected. This ensures that the sample is representative and minimizes bias. Common probability sampling methods include:
In non-probability sampling, not every member of the population has a known or equal chance of being included in the sample, which may lead to bias. These methods are often used in exploratory research or when probability sampling is not feasible. Common non-probability methods include:
Â